With Latimer, an LLM that includes black and brown voices, data is still king
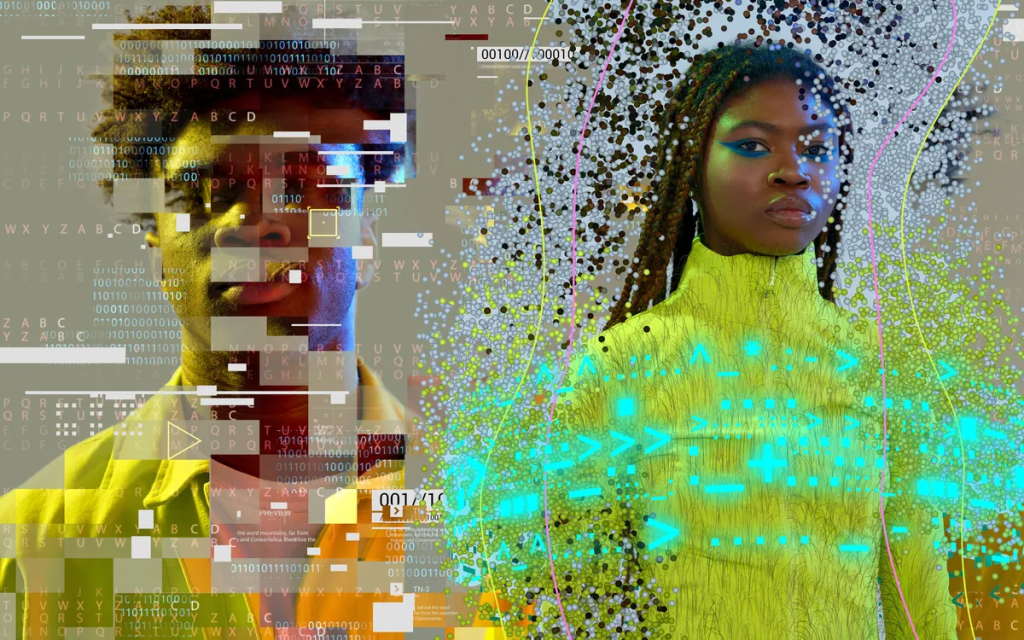
AI’s inclusivity problem is no secret. According to the ACLU, AI systems can perpetuate housing discrimination and bias in the justice system, among other harms. Bias in the data an AI model relies on is reproduced in its results.
Large Language Models (LLMs) share this problem; they can reproduce bias in medical settings and perpetuate harmful stereotypes, among other problems. To combat that, the New York City–based FutureSum AI is building Latimer, the first “racially inclusive large language model.” Latimer—named after a pioneering engineer of the 19th and early 20th centuries—hopes to reduce bias, better represent underrepresented voices, and prevent results that erase or minimize black and brown cultural data.
Hugging Face, the hub for the open-source AI community, lists over 2,700 “conversational” AI models.
“Data is king,” says Malur Narayan, technology advisor for FutureSum AI. “The only way to create a moat is to have the relevant data for the topic you’re trying to address.”
Curating a Different Dataset
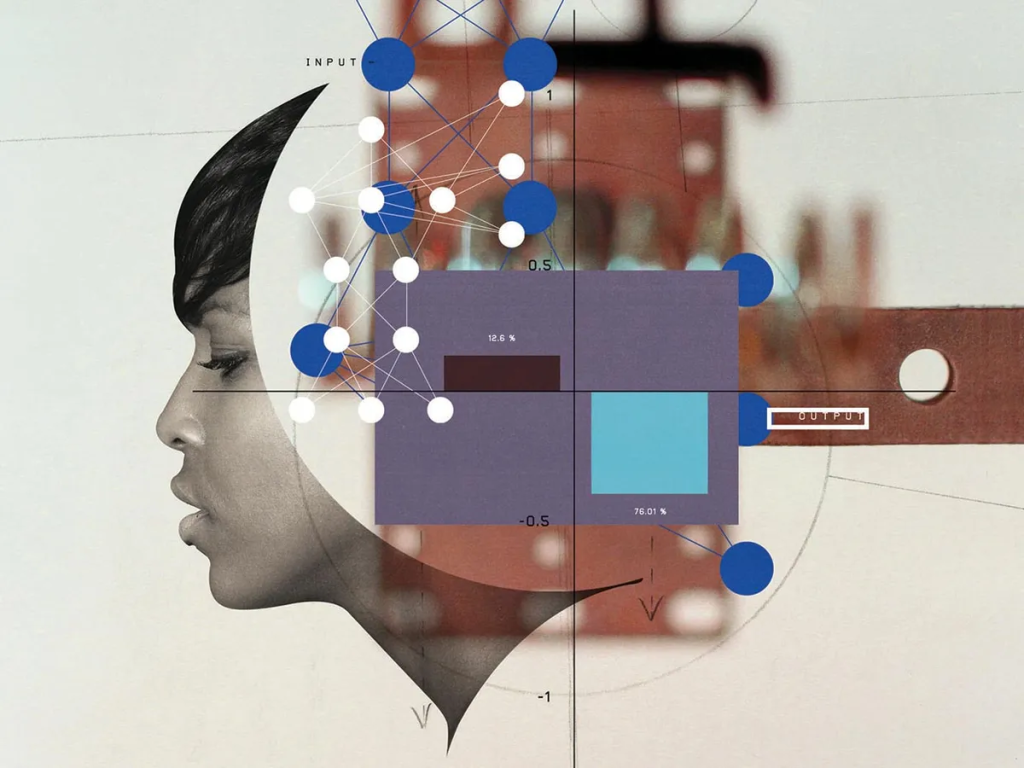
Large Language Models have proliferated with incredible speed. Hugging Face, the hub for the open-source AI community, lists over 2,700 “conversational” AI models. Yet most are trained on similar data (Common Crawl is a popular source), and many user-facing apps that use an LLM lean on one of several large providers, such as OpenAI and Anthropic. In other words, the vast majority of the AI apps and tools popular right now are rooted in a handful of models trained on similar data.
Latimer also leans on a popular LLM provider (it uses OpenAI’s ChatGPT as its foundation model) but augments that model with additional data to better represent minority voices. The company has an exclusive partnership with New York Amsterdam News, a black-owned newspaper founded in 1909, and works with historically black colleges and universities to obtain access to both license-free and licensed data.
“We’re going for any and all available sources and resources, which we believe are more representative and more accurate sources, based on our own judgement, and a set of criteria we use to determine legitimacy,” says Narayan. Latimer’s data has a particular focus on educational and academic sources, as “they’re more likely to be legitimate sources.” With these sources available, Latimer’s engineers can use weighting techniques to adjust the model’s weights to counteract any known biases.
Narayan says FutureSum isn’t ready to release benchmark results for Latimer yet. But, he adds, the organization hopes to have some available within weeks. The LLM is currently in beta testing and announced its public wait list on 24 January. Those who sign up for the wait list will join students from Alabama’s Miles College in testing the model.
A New Kind of Generation
At its heart, Latimer relies on a technique known as retrieval-augmented generation (RAG). This technique was first described in a 2020 paper from researchers at Meta in collaboration with the University College London and New York University. RAG makes it possible for LLMs to verify and update their knowledge by accessing and cross-referencing a second source of data.
RAG inspired a major shift in how the world’s best LLMs function, Narayan says. It can improve an LLM’s accuracy, help it find and cite a source for data it provides in its response, or unlock access to new data that wasn’t available when the model was trained. IBM offers it as a feature of its Watsonx.ai platform; Microsoft and OpenAI use something like it to present Bing search results in Co-Pilot; OpenAI uses something like it to allow for custom GPTs that reference files provided by users.
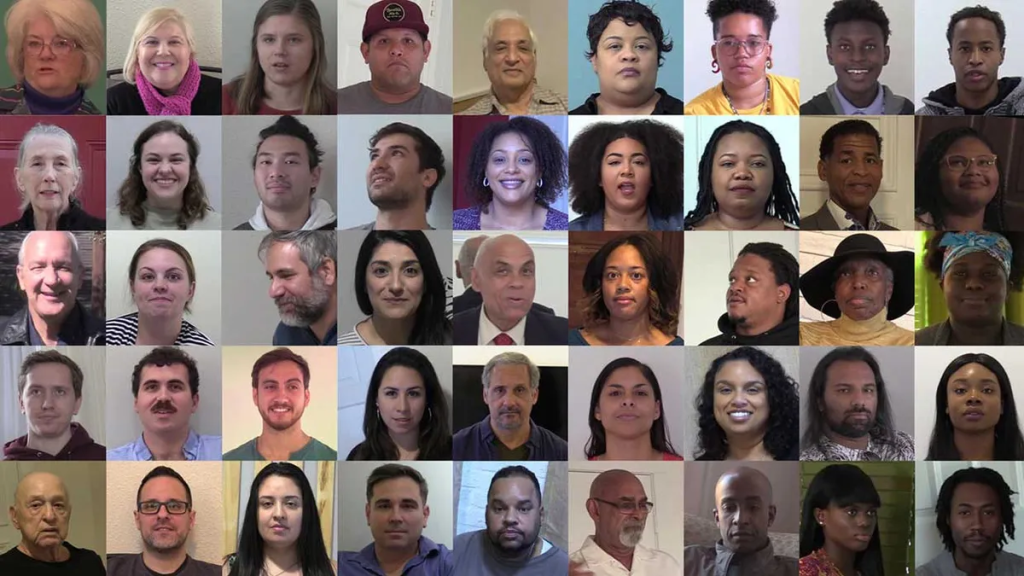
“We’re developing an API, but the most important thing is the key applications. We want to help pharmaceutical companies have better reach into this community for clinical trials, help recruiters attract a black audience, help banking, and finance, and insurance.”—MALUR NARAYAN, FUTURESUMAI
Latimer specifically uses RAG as a lens to focus its ability to detect bias and promote underrepresented voices. “We’re using that not just for recent information, but also to ensure the data itself is more comprehensive when it comes to the topic we’re addressing, “says Narayan. “When a prompt is sent by a user, it first goes into our RAG model to see if that topic is relevant.” That includes preprompting rules to ensure responses are “more accurate and relevant to black history, black culture, and black heritage, and that there’s minimal bias.”
The approach is sound in theory, but it’s important to check that it works in practice. Narayan says Latimer’s early testing was mostly conducted through manual human feedback including A/B comparisons between its performance and that of the most popular LLMs, such as ChatGPT and Bard. Manual testing is difficult to scale, however, so the company also relies on automated bias-detection tools and comparisons with fairness metrics. This, in part, is what Latimer’s public beta test should help establish, Narayan says, as more users will provide more responses to examine.
Once testing is complete, Latimer plans to provide an API that any company or organization can use to tap into the LLM. It’s an obvious move from both a technical and business perspective; many organizations offering a commercial LLM eventually offer an API to let developers access it for a fee. For Latimer, however, it’s ultimately about the fulfillment of Latimer’s purpose.
“We’re developing an API, but the most important thing is the key applications. We want to help pharmaceutical companies have better reach into this community for clinical trials, help recruiters attract a black audience, help banking, and finance, and insurance,” says Narayan. “That’s our end goal. How do we let businesses better communicate with a black audience, or a brown audience.”